IBM Watson has come with an intelligent Visual Recognition API which can identify the objects on the image, human face according to the training sets has been provided which helps us to build our own custom classes without even worrying about the algorithm.
Visual Recognition API API provides developers to develop their own application with preferred programming language. Watson Visual Recognition provides API support for JAVA, Node.js, Python and also REST API access.
I will walk you through the Watson Visual Recognition API,
- How to train the API and create custom classifier?
- How to identify the human faces using the Visual Recognition API?
Get started with clicking any 2 image sets of faces with at least 10 images of each faces, and zip those images with respective names.
Hardware Required
- Raspberry Pi 3
- Picamera
Web Component Setup
Walk through to setup and get key for IBM Watson Visual Recognition API,- Register for IBM Bluemix with US South or your preferred region.
- Login to the IBM Bluemix.
- Select Create app, to start with the new applet
- Find Watson on Services tab
- On Watson services, select Visual Recognition API
- Click on create to get started.
- On Service credentials, select on credential-1 and copy the api-key
Install Watson Visual Recognition Python SDK
Official SDK Repo: GithubTo install, use
pip
or easy_install
:$ pip install --upgrade watson-developer-cloud
or
$ easy_install --upgrade watson-developer-cloud
Train a Custom Classifier
- Clone the source code using,
git clone https://github.com/suryasundarraj/watson-visual-recognition-raspberry-pi-camera.git
- Get into the folder
cd watson-visual-recognition-raspberry-pi-camera
- Move the zip files made earlier to the current folder
- Update the file names on the code,
face_zip_1 = "" face_zip_2 = ""
- Update the api-key from the IBM Watson Visual Recognition Service Credentials on train.py and face.py
- Run the Script,
python train.py
- Wait for the classifier id to get generated
- Replace the classifier id to the face.py,
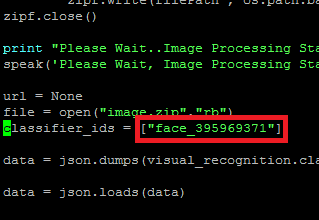
Install Picamera
- From the prompt, run "sudo raspi-config". If the "camera" option is not listed, you will need to run a few commands to update your Raspberry Pi. Run "sudo apt-get update" and "sudo apt-get upgrade"
- Run "sudo raspi-config" again - you should now see the "camera" option.
- Navigate to the "camera" option, and enable it. Select “Finish” and reboot your Raspberry Pi.
Run Face Recognition
- Make sure you update the api-key on face.py and train.py
- Connect the picamera to the rpi
- Change the name on the face.py with your name,
- Run the script, and rpi camera starts capturing your image and uploads to the watson api.
python face.py
- Watson api, returns json data with the score for the matches.
- Based on the high score, the program decides the face on the classifier.
If there is no matches api responds,
IBM Visual Recognition service allows only one custom classifier instance for free account, if you decide to play more with the api. Check out the pricing plans on the service page.
With this, You will be able to use the visual recognition api with human face recognition. Feel free to comment below if you are looking for some help and also please share your experiences or any particular challenges that you might have faced in executing this setup. I will be back soon with some more interesting demos.
Thank you for posting such a great blog. I found your website perfect for my needs. Read About Facial Recognition Software
ReplyDelete